
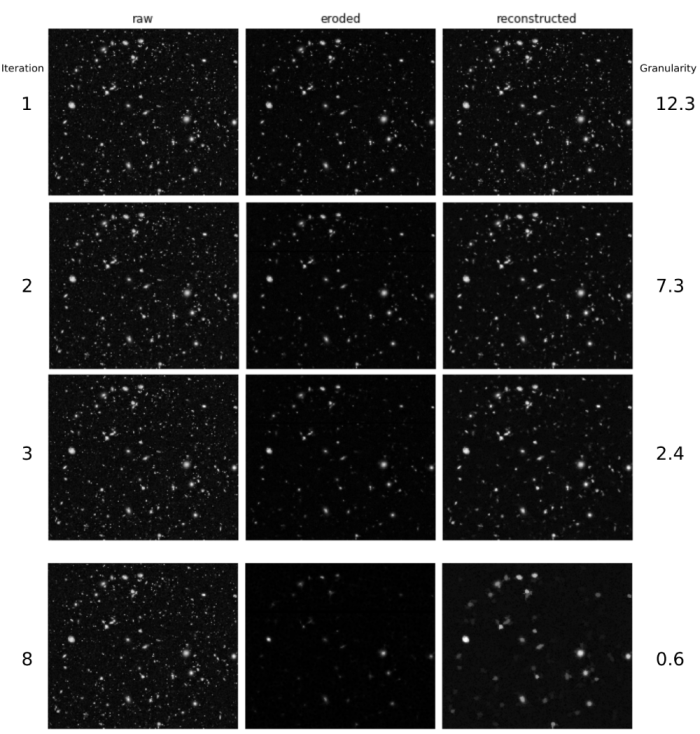
Finally, algorithms are usually configured using a few-hopefully representative-images from the experiment, but variations in signal quality and the presence of noise pose challenges to the robustness and reliability of the solution at large scale. Second, the most popular solutions for nucleus segmentation were originally formulated and adopted several decades ago when the biological systems and phenotypes of interest were often simpler however, as biology pushes the limits of high-throughput cellular and tissue models, natural and subtle variations of biologically meaningful phenotypes are more challenging to segment. First, existing algorithms have limitations due to the assumptions made in the computational design that do not always hold, such as thresholding methods that assume bimodal intensity distributions, or region growing that expects clearly separable boundaries. There are several causes for segmentation errors.
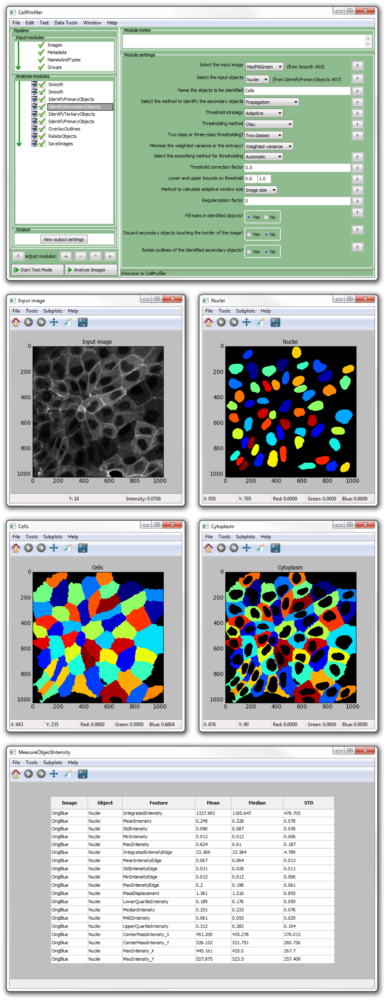
These may silently propagate to downstream analyses, yielding unreliable measures or systemic noise that is difficult to quantify and factor out.
#Cellprofiler analyzing multiple images software
Many of these strategies are readily available in various bioimage software packages 11, including open source options such as CellProfiler 12, Ilastik 10, and ImageJ/Fiji 13, facilitating their adoption in routine biological research.ĭespite widespread adoption, segmentation tools in biology generally do yield nontrivial amounts of segmentation error. Machine learning-based segmentation methods have also been introduced for segmenting cells 10, which typically require annotated examples in the form of segmentation masks or interactive scribbles. One of the most commonly used strategies for nucleus segmentation is Otsu's thresholding method 7 followed by seeded watershed 8, 9, because of its effectiveness, simplicity of use and computational efficiency. One critical step in quantifying fluorescence images is often the identification of the nucleus of each cell with a DNA stain, and there is a long history of research efforts to design and improve nuclear and cellular segmentation 6. Accurate and automated analysis methods are key to successfully quantify relevant biology in such large image collections. The most widely used quantitative imaging technique in biological laboratories is fluorescence imaging with automation it can easily produce terabytes of primary research data 5. From image-based assays to high-content screening 1, 2, microscopy images have led to understanding genetic perturbations, pursuing drug discovery, and phenotyping cells in biomedical applications and cell biology research 3, 4. Image analysis is a powerful tool in cell biology to collect quantitative measurements in time and space with precision, speed, and sensitivity.
